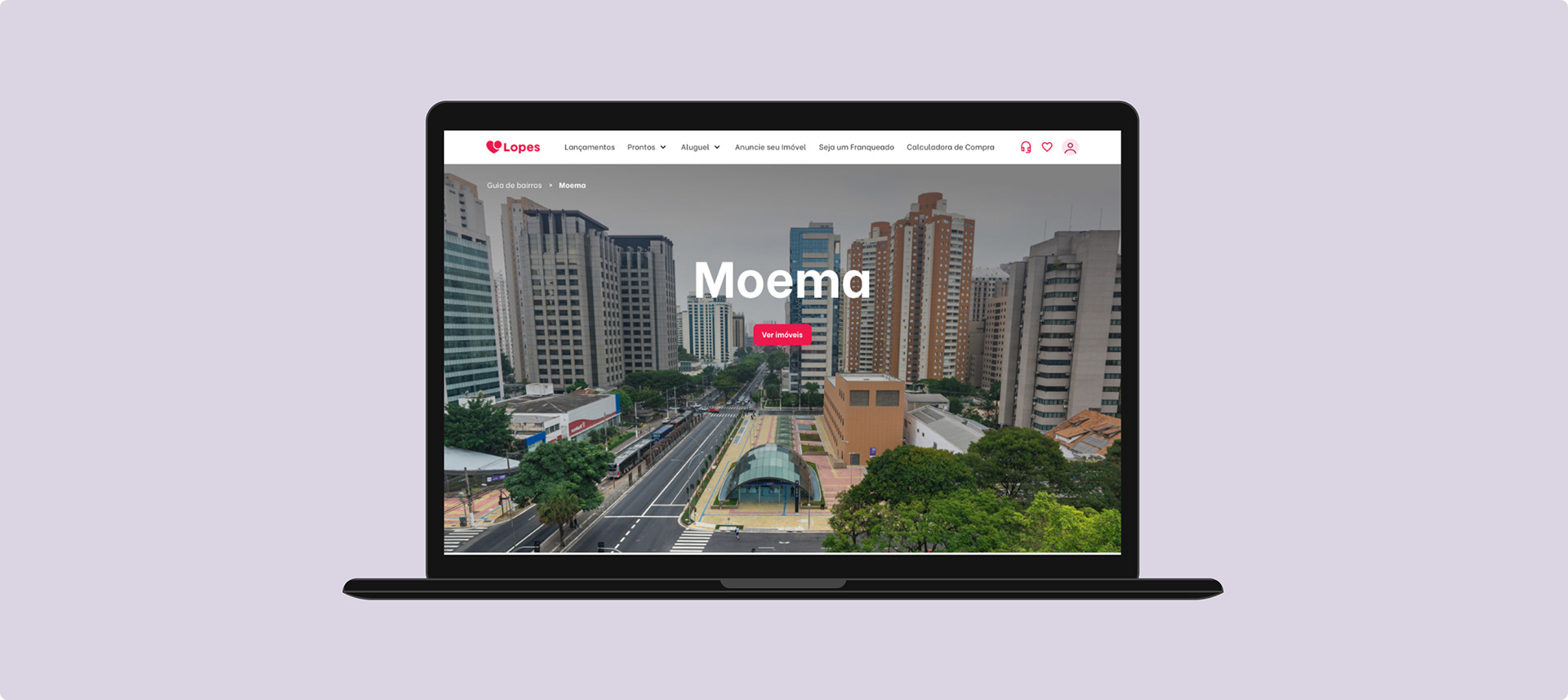
What I did
My Role: Senior Product Designer
Year: 2025
Skills Applied: UX Research, UI design, Usability Testing, AI & Front-end
Tools: Figma, Miro, ChatGPT, Bolt
Overview
Real estate platforms are overloaded with listings but often lack contextual insights about neighborhoods, a key pain point for decision-making in both renting and buying.
Lopes, a leading real estate company in Brazil, identified an opportunity to transform its Neighborhood Guide into a trust-building and conversion-boosting experience through AI-generated content and low-code prototyping.
The goal was to validate a scalable design model that emphasized trust, clarity, and user engagement, and could be expanded across the entire country.
Problem
Users struggled to find reliable and intuitive information about neighborhoods. Pages were static, lacked personalization, and didn’t support decision-making beyond property specs.
Internally, Lopes faced long development cycles for updates and difficulty scaling UX improvements across its regional pages.
There was also significant internal pressure to launch quickly, as major competitors were consistently ranking higher on Google search results when users looked for information about neighborhoods. This visibility gap was impacting Lopes' online discoverability and positioning as a reliable source for real estate insights.
Traditional development for a project of this scale was estimated to take up to 6 months. Given the urgency to validate a scalable experience and deliver value faster, we opted to launch Lopes’ first fully AI and low-code powered product, an approach that significantly reduced delivery time and enabled rapid iteration.
Key Challenges:
• A fragmented user experience with low engagement.
• Absence of interactive tools and localized insights.
• Slow iteration cycle for experimentation and testing.
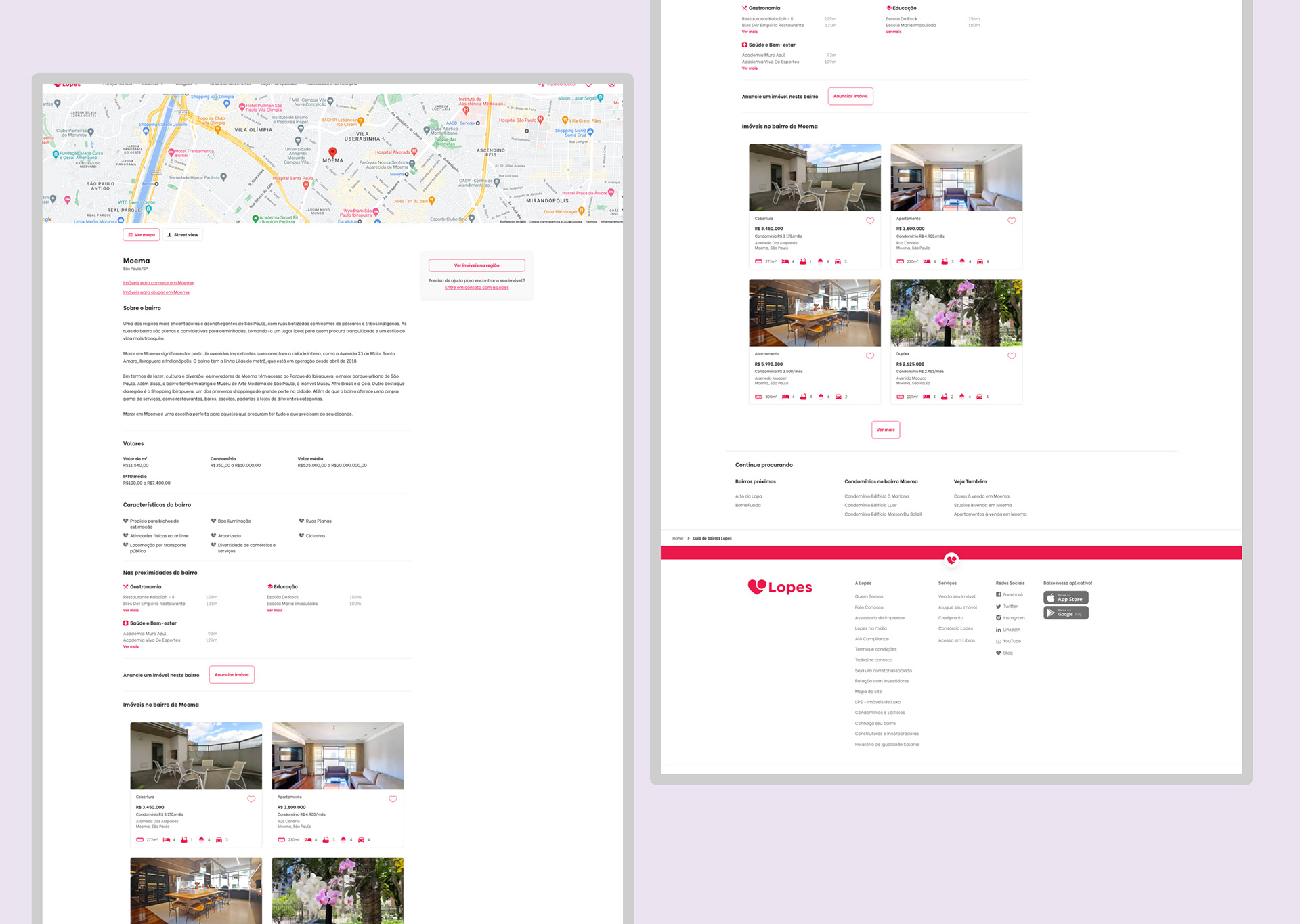
Previous version of the Neighborhood Guide. The layout lacked contextual information, interactivity, and user-centric content, contributing to low engagement and limited decision-making support.
Process
Users struggled to find reliable and intuitive information about neighborhoods. Pages were static, lacked personalization, and didn’t support decision-making beyond property specs.
Internally, Lopes faced long development cycles for updates and difficulty scaling UX improvements across its regional pages.
There was also significant internal pressure to launch quickly, as major competitors were consistently ranking higher on Google search results when users looked for information about neighborhoods. This visibility gap was impacting Lopes' online discoverability and positioning as a reliable source for real estate insights.
Traditional development for a project of this scale was estimated to take up to 6 months. Given the urgency to validate a scalable experience and deliver value faster, we opted to launch Lopes’ first fully AI and low-code powered product, an approach that significantly reduced delivery time and enabled rapid iteration.
Key Challenges:
• A fragmented user experience with low engagement.
• Absence of interactive tools and localized insights.
• Slow iteration cycle for experimentation and testing.
User Research & Personas
• Based on real customer interviews and analytics, three core profiles were defined: investors, families, and young professionals.
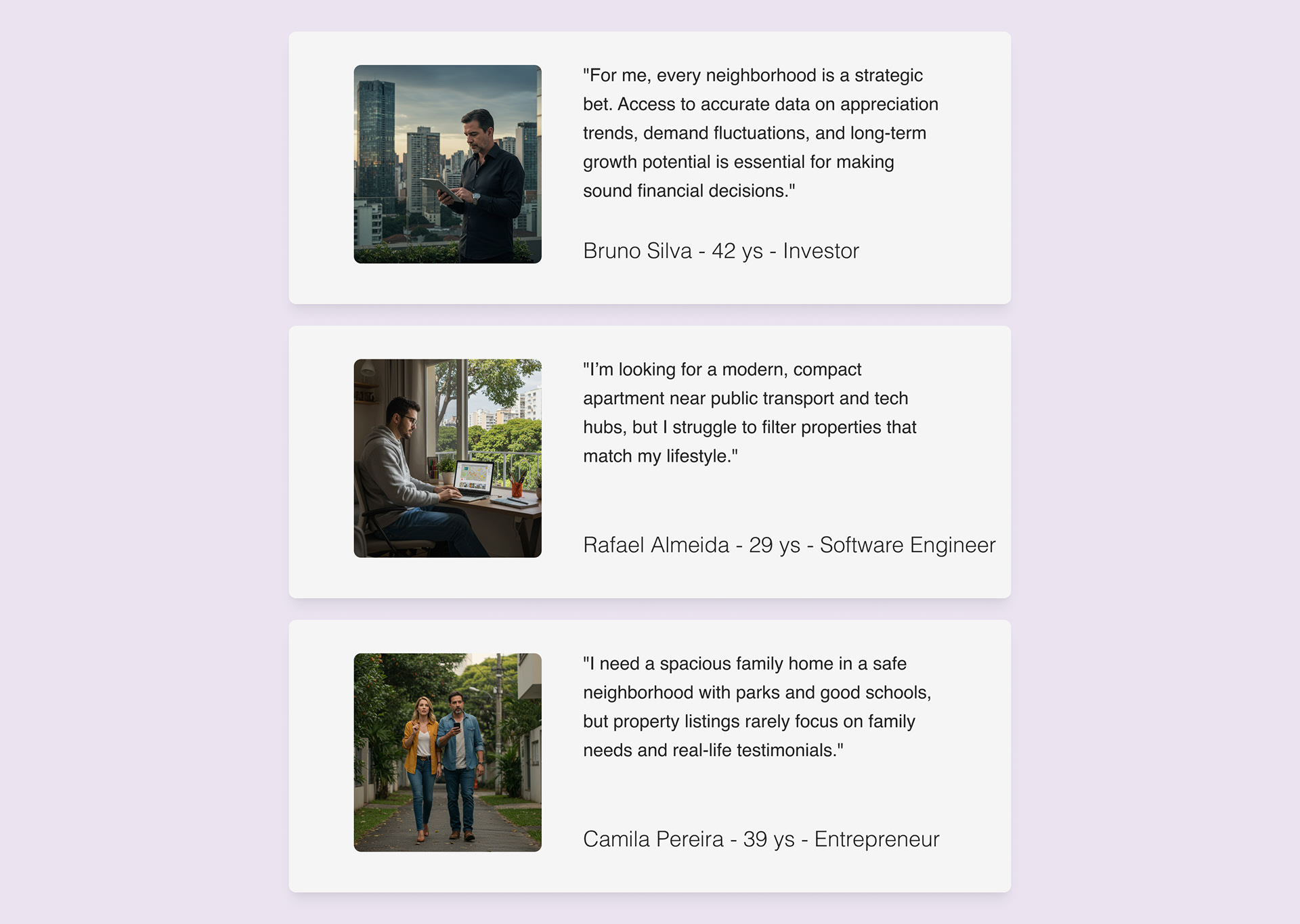
Key personas identified through research, including investors, families, and young professionals, helped shape the redesigned user journey.
Competitor Benchmarking
• A comprehensive analysis of national and international real estate platforms highlighted UX and content strategy gaps that Lopes could address.
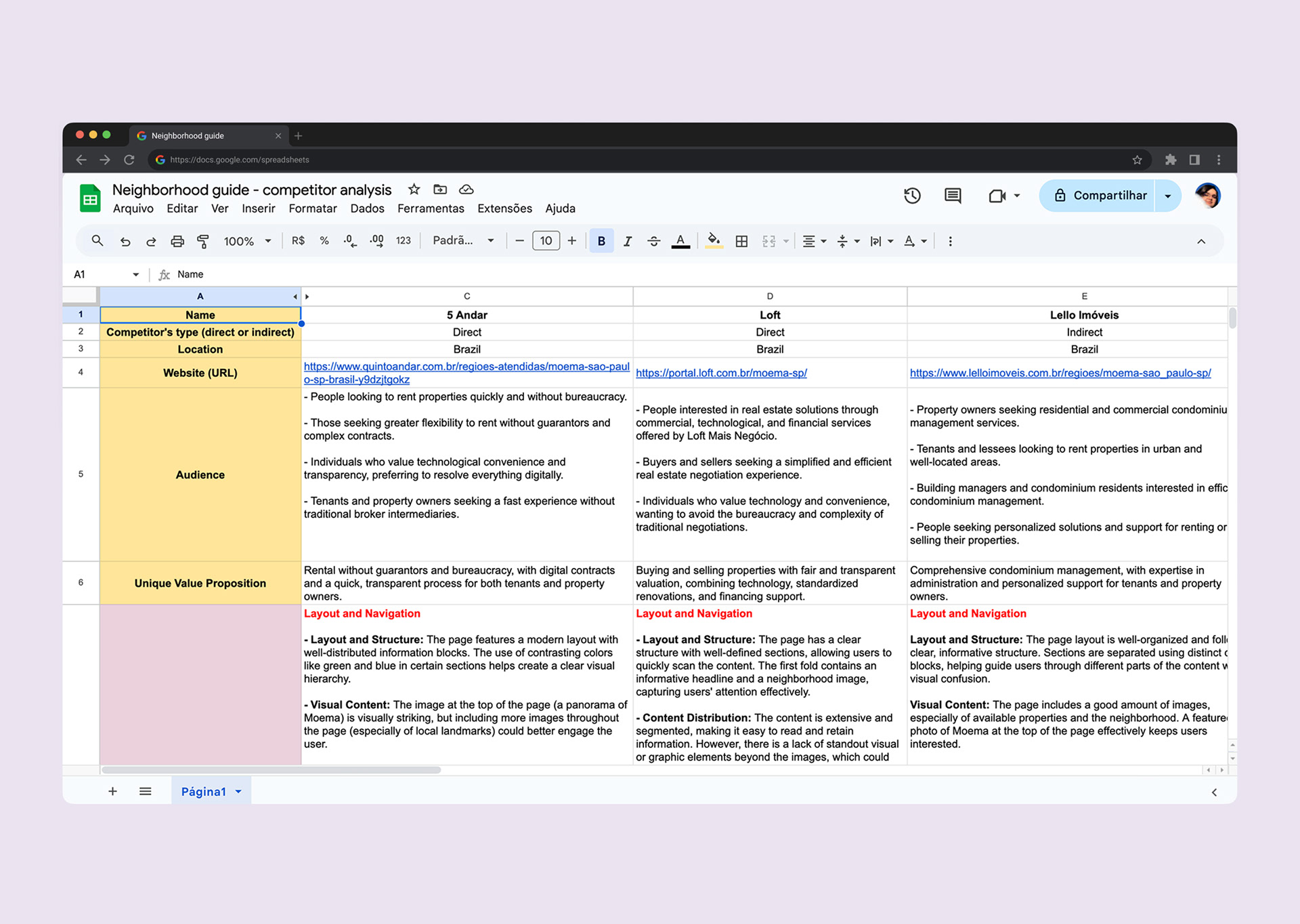
Competitive benchmarking revealed UX and feature gaps across national and international real estate platforms.
Journey Mapping
• User flows and emotional journeys were mapped to identify friction points and decision-making triggers along the real estate funnel.
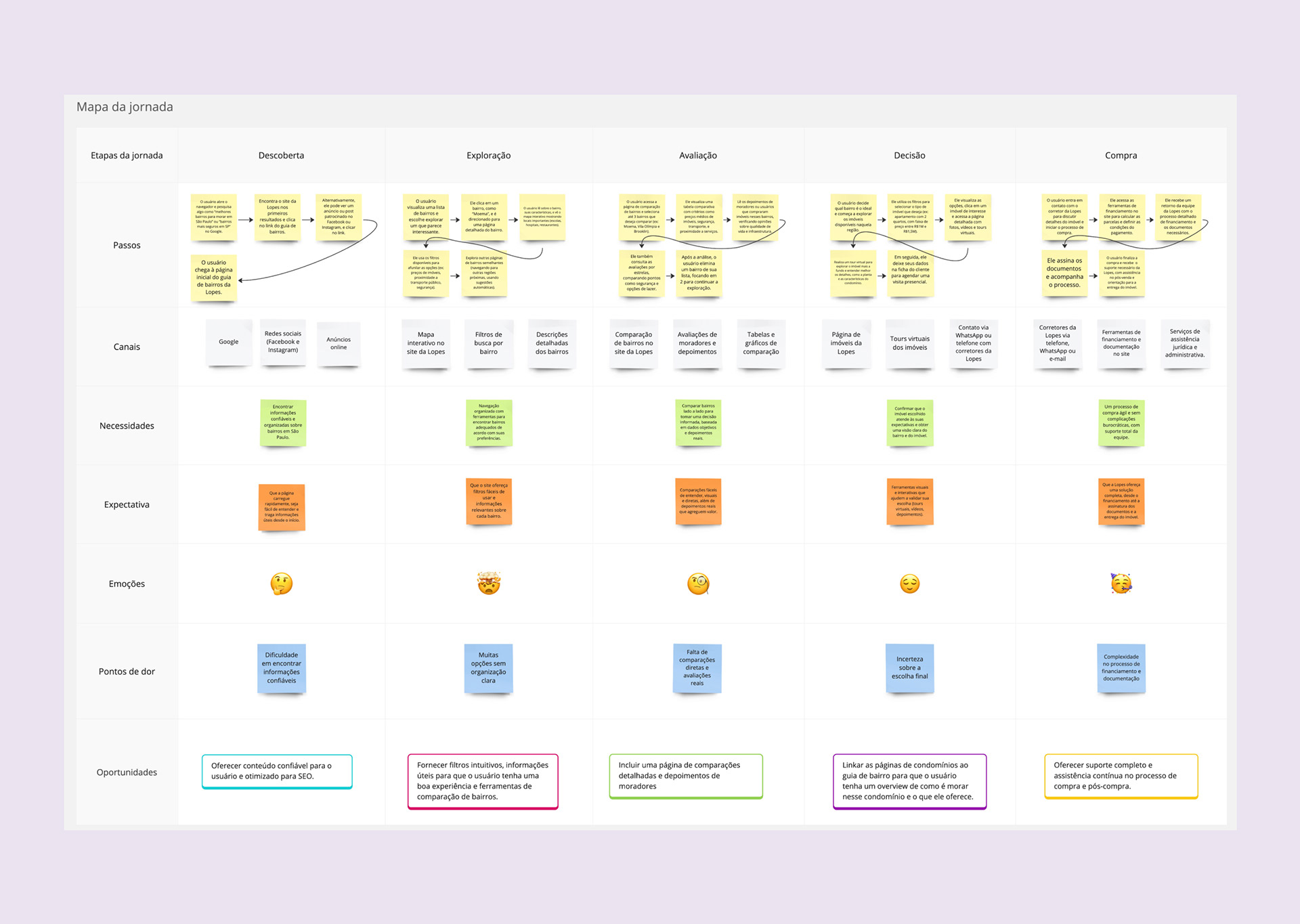
Mapped user flows for each audience segment, identifying friction points across discovery, evaluation, and decision-making phases.
AI & Low-Code Stack Implementation
To support scale and speed, we used:
• AI-assisted tools to help structure and streamline neighborhood data and content generation.
• Low-code platforms to assemble and deploy interactive components, allowing for faster iteration and testing.
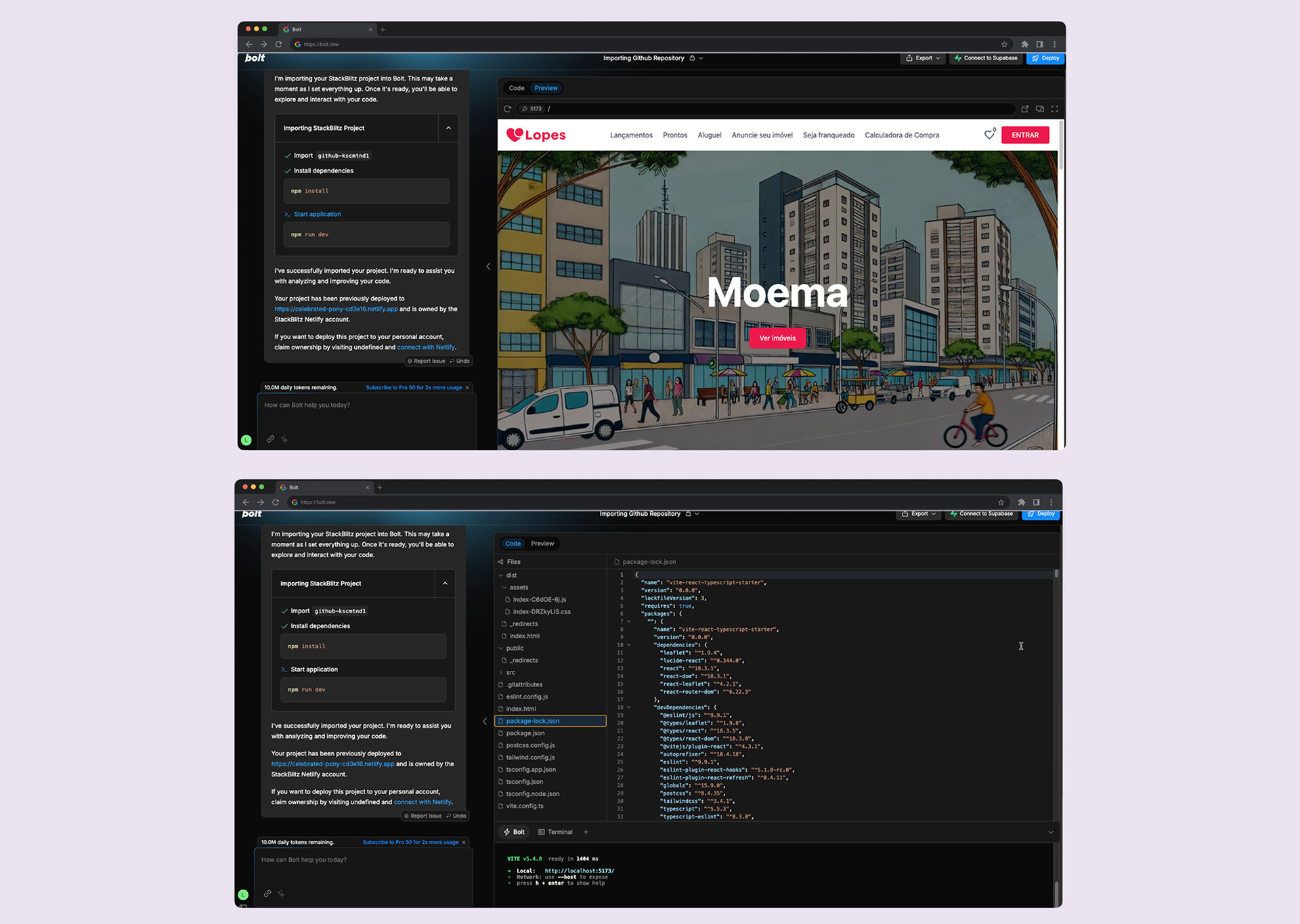
Bolt was the low-code platform used to build and launch the first version of the Neighborhood Guide.
Solution
A redesigned platform focused on clarity, trust, and content relevance.
Traditionally, a rollout of this scale would have required up to 6 months of development time. Leveraging AI and low-code, we successfully launched the first version of the new Neighborhood Guide in just 2 months.
Core Enhancements (V0):
• Structured neighborhood descriptions enhanced by AI-assisted content generation.
• Static neighborhood maps highlighting boundaries and context.
• Clear content hierarchy for improved readability and SEO.
• Cards displaying featured listings within each neighborhood.
• Internal linking to nearby neighborhoods and related listings.
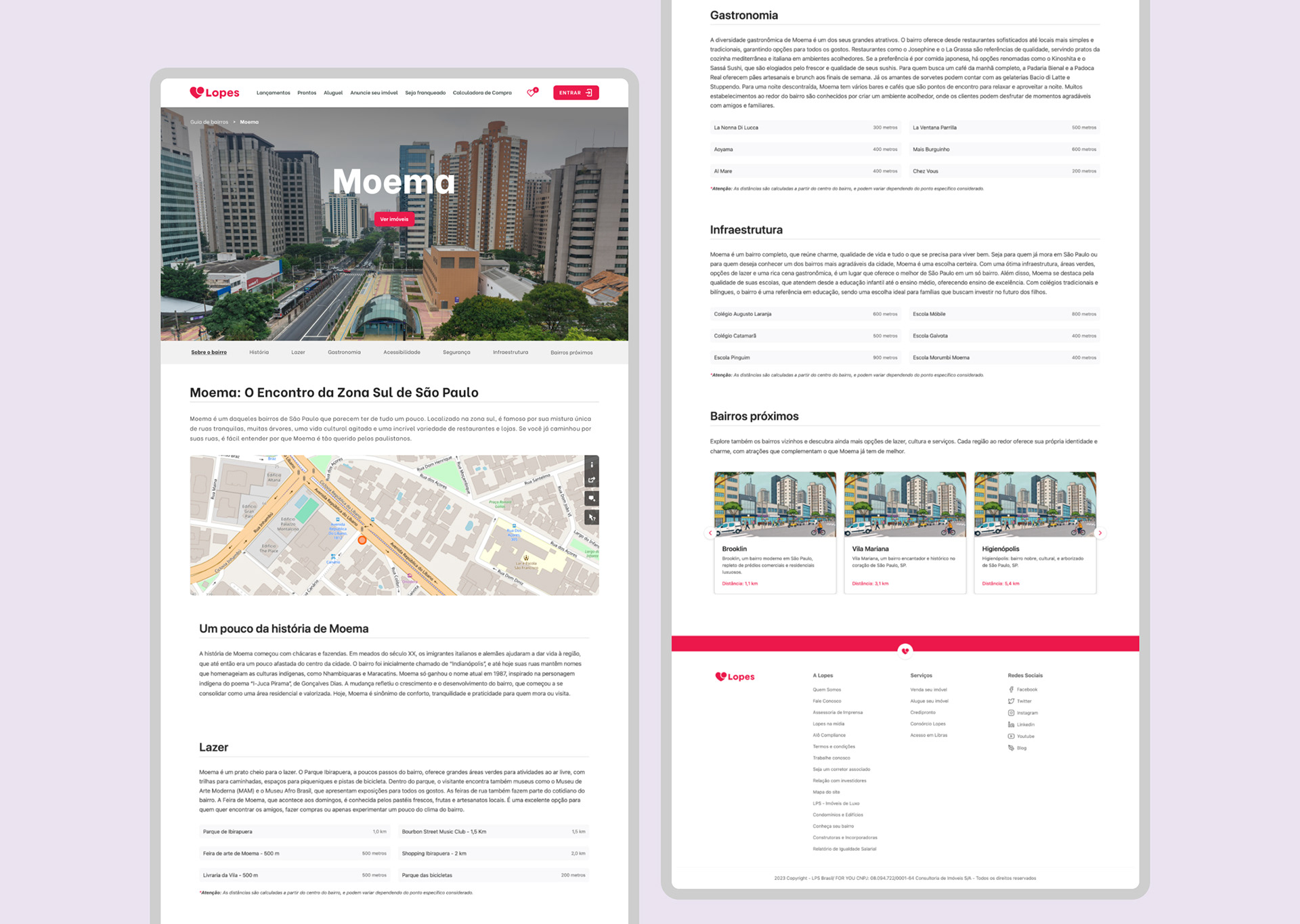
V0 of the Neighborhood Guide. Due to the short timeline and our first-time use of the Bolt low-code platform, we prioritized a simpler implementation than originally envisioned in the wireframes, focusing on core content delivery and structured layout.
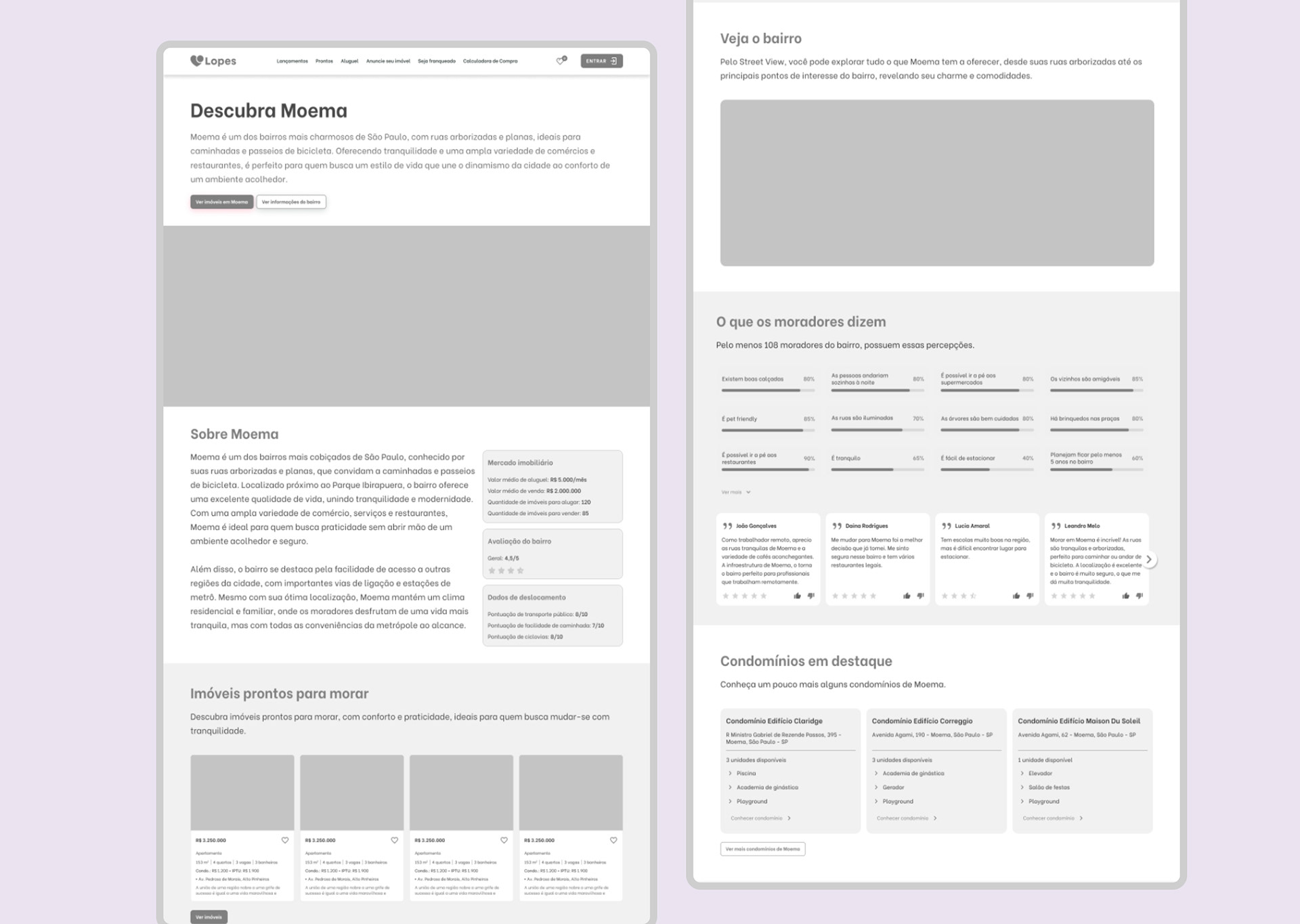
Wireframe showcasing the full experience vision for future iterations of the Neighborhood Guide. Designed to include advanced interactivity and richer neighborhood context as the product evolves beyond its initial low-code MVP.
Impact
The initial rollout included more than 40 neighborhoods, with Moema featured as one of the key examples.
The new version of the Neighborhood Guide is live and operational, and its impact is being actively monitored through user behavior and analytics.
Key Outcomes:
• Greater internal alignment and momentum around content-driven UX.
• Product launched in record time for the company: delivered in just 2 months, a 65% reduction in time compared to the traditional 6-month development cycle, enabled by AI and low-code tools.
• Framework and structure built to scale the model to all neighborhoods, using AI and low-code tools.
Learnings
• AI is not just content automation; it’s a UX enabler when paired with smart data structuring.
• Low-code tools significantly accelerated our testing and iteration process, allowing us to validate hypotheses at a fraction of the traditional time.
• Strategic UX is about removing friction and adding relevance. By designing for both trust and transaction, we turned a static real estate page into a guided digital experience.